Detection of fraudulent companies
Problem Statement:
The mission of the Financial Crime Investigation Service (FNTT, Lithuania) is to protect the state financial system by revealing criminal actions and other violations of law. The Service pursues this through a range of operational measures, conducting pre-trial investigation and prevention of criminal acts, taking measures for combating money laundering, for warrantying the legitimacy in the receipt and use of the financial support funds of the EU and other countries, checking the commercial and financial activity of organisations and searching for tax evasion cases, expecting that this activity would influence as much as possible the actual revenue payable to the state budget.
Current priorities of FNTT are:
- international and domestic criminal acts related with the fraud and avoidance of value added tax (VAT), excise duties, income tax and other taxes as well as fraudulent management of accounts;
- criminal acts related to the illegal obtaining and use of EU support funds;
- legalisation of the proceeds of crime (money laundering) and illicit enrichment;
- criminal acts in the field of swindling and misappropriation or squandering of property in such financial institutions as banks and credit unions and manipulating the prices (fraud) in the energy sector.
One of the most important factors in work of FNTT is detection of potentially fraudulent companies, often established or acquired for illegal purposes. There is a number of potential indicators for such companies, such as drastic change of turnover, owners, etc. However, it is not easy to collect data about all the companies and identify risky ones. To make this process more efficient FNTT needs instruments, such as:
- Tools for extracting usable and interoperable information from heterogeneous Big Data sources.
- New predictive analysis models for trend detection and probability prediction of financial crime activities using Machine Learning methods.
- New Human-Machine Interaction and visual analytics techniques, integrating search and visualisation capabilities in support of everyday analyst’s tasks.
Moreover, there are emerging threats that could make the abovementioned analyses in the future even more difficult. For example, an arising challenge could be solutions like SonicVortex Transactions (https://www.ccn.com/soniccoin/) that can be treated as a next-generation crypto currency platform. It enables hiding encrypted bitcoin transactions in innocent multimedia data and offers a stealthy address and built-in TOR support. This can potentially provide tremendous difficulties for investigating financial crimes. Such concepts may hinder effective financial analyses, thus they need to be addressed.
In the case study, a set of methods and a prototype for collecting data from various sources and identifying potentially fraudulent individuals or companies will be built. The companies/individuals, potentially involved in financial fraud, such as money laundering, VAT avoidance, fraud in public procurement and illicit use of EU funds will be identified. Then, the collected data will be merged (fused) with their internal data sources, and, based on the further investigation, financial crime will be prevented or identified. Such use case will allow to test usability of the methods and prototypes, and will enable FNTT to embrace OSINT and AI.
Partners to be envolved:


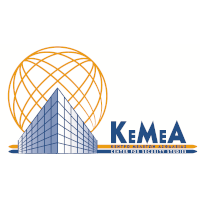
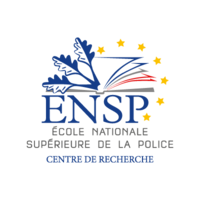